python实现Canny与Hough算法
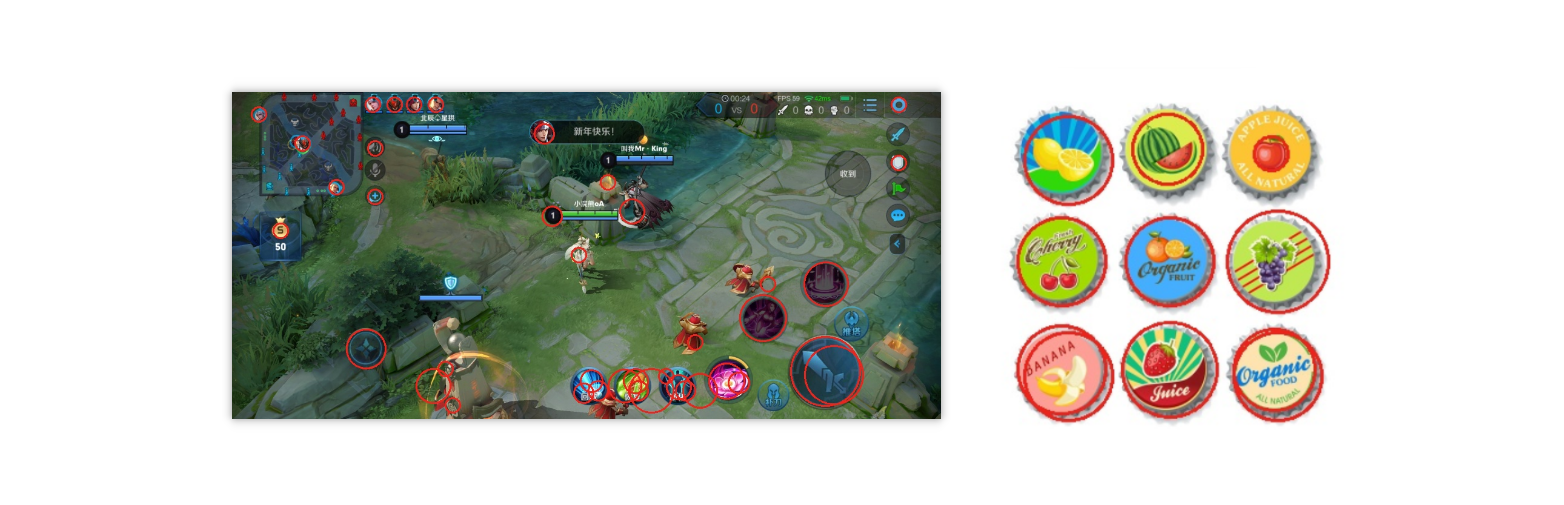
Contents
任务说明:编写一个钱币定位系统,其不仅能够检测出输入图像中各个钱币的边缘,同时,还能给出各个钱币的圆心坐标与半径。
效果
1 代码实现
# Author: Ji Qiu (BUPT)
# filename: my_canny.py
import cv2
import numpy as np
class Canny:
def __init__(self, Guassian_kernal_size, img, HT_high_threshold, HT_low_threshold):
'''
:param Guassian_kernal_size: 高斯滤波器尺寸
:param img: 输入的图片,在算法过程中改变
:param HT_high_threshold: 滞后阈值法中的高阈值
:param HT_low_threshold: 滞后阈值法中的低阈值
'''
self.Guassian_kernal_size = Guassian_kernal_size
self.img = img
self.y, self.x = img.shape[0:2]
self.angle = np.zeros([self.y, self.x])
self.img_origin = None
self.x_kernal = np.array([[-1, 1]])
self.y_kernal = np.array([[-1], [1]])
self.HT_high_threshold = HT_high_threshold
self.HT_low_threshold = HT_low_threshold
def Get_gradient_img(self):
'''
计算梯度图和梯度方向矩阵。
:return: 生成的梯度图
'''
print ('Get_gradient_img')
new_img_x = np.zeros([self.y, self.x], dtype=np.float)
new_img_y = np.zeros([self.y, self.x], dtype=np.float)
for i in range(0, self.x):
for j in range(0, self.y):
if j == 0:
new_img_y[j][i] = 1
else:
new_img_y[j][i] = np.sum(np.array([[self.img[j - 1][i]], [self.img[j][i]]]) * self.y_kernal)
if i == 0:
new_img_x[j][i] = 1
else:
new_img_x[j][i] = np.sum(np.array([self.img[j][i - 1], self.img[j][i]]) * self.x_kernal)
gradient_img, self.angle = cv2.cartToPolar(new_img_x, new_img_y)#返回幅值和相位
self.angle = np.tan(self.angle)
self.img = gradient_img.astype(np.uint8)
return self.img
def Non_maximum_suppression (self):
'''
对生成的梯度图进行非极大化抑制,将tan值的大小与正负结合,确定离散中梯度的方向。
:return: 生成的非极大化抑制结果图
'''
print ('Non_maximum_suppression')
result = np.zeros([self.y, self.x])
for i in range(1, self.y - 1):
for j in range(1, self.x - 1):
if abs(self.img[i][j]) <= 4:
result[i][j] = 0
continue
elif abs(self.angle[i][j]) > 1:
gradient2 = self.img[i - 1][j]
gradient4 = self.img[i + 1][j]
# g1 g2
# C
# g4 g3
if self.angle[i][j] > 0:
gradient1 = self.img[i - 1][j - 1]
gradient3 = self.img[i + 1][j + 1]
# g2 g1
# C
# g3 g4
else:
gradient1 = self.img[i - 1][j + 1]
gradient3 = self.img[i + 1][j - 1]
else:
gradient2 = self.img[i][j - 1]
gradient4 = self.img[i][j + 1]
# g1
# g2 C g4
# g3
if self.angle[i][j] > 0:
gradient1 = self.img[i - 1][j - 1]
gradient3 = self.img[i + 1][j + 1]
# g3
# g2 C g4
# g1
else:
gradient3 = self.img[i - 1][j + 1]
gradient1 = self.img[i + 1][j - 1]
temp1 = abs(self.angle[i][j]) * gradient1 + (1 - abs(self.angle[i][j])) * gradient2
temp2 = abs(self.angle[i][j]) * gradient3 + (1 - abs(self.angle[i][j])) * gradient4
if self.img[i][j] >= temp1 and self.img[i][j] >= temp2:
result[i][j] = self.img[i][j]
else:
result[i][j] = 0
self.img = result
return self.img
def Hysteresis_thresholding(self):
'''
对生成的非极大化抑制结果图进行滞后阈值法,用强边延伸弱边,这里的延伸方向为梯度的垂直方向,
将比低阈值大比高阈值小的点置为高阈值大小,方向在离散点上的确定与非极大化抑制相似。
:return: 滞后阈值法结果图
'''
print ('Hysteresis_thresholding')
for i in range(1, self.y - 1):
for j in range(1, self.x - 1):
if self.img[i][j] >= self.HT_high_threshold:
if abs(self.angle[i][j]) < 1:
if self.img_origin[i - 1][j] > self.HT_low_threshold:
self.img[i - 1][j] = self.HT_high_threshold
if self.img_origin[i + 1][j] > self.HT_low_threshold:
self.img[i + 1][j] = self.HT_high_threshold
# g1 g2
# C
# g4 g3
if self.angle[i][j] < 0:
if self.img_origin[i - 1][j - 1] > self.HT_low_threshold:
self.img[i - 1][j - 1] = self.HT_high_threshold
if self.img_origin[i + 1][j + 1] > self.HT_low_threshold:
self.img[i + 1][j + 1] = self.HT_high_threshold
# g2 g1
# C
# g3 g4
else:
if self.img_origin[i - 1][j + 1] > self.HT_low_threshold:
self.img[i - 1][j + 1] = self.HT_high_threshold
if self.img_origin[i + 1][j - 1] > self.HT_low_threshold:
self.img[i + 1][j - 1] = self.HT_high_threshold
else:
if self.img_origin[i][j - 1] > self.HT_low_threshold:
self.img[i][j - 1] = self.HT_high_threshold
if self.img_origin[i][j + 1] > self.HT_low_threshold:
self.img[i][j + 1] = self.HT_high_threshold
# g1
# g2 C g4
# g3
if self.angle[i][j] < 0:
if self.img_origin[i - 1][j - 1] > self.HT_low_threshold:
self.img[i - 1][j - 1] = self.HT_high_threshold
if self.img_origin[i + 1][j + 1] > self.HT_low_threshold:
self.img[i + 1][j + 1] = self.HT_high_threshold
# g3
# g2 C g4
# g1
else:
if self.img_origin[i - 1][j + 1] > self.HT_low_threshold:
self.img[i + 1][j - 1] = self.HT_high_threshold
if self.img_origin[i + 1][j - 1] > self.HT_low_threshold:
self.img[i + 1][j - 1] = self.HT_high_threshold
return self.img
def canny_algorithm(self):
'''
按照顺序和步骤调用以上所有成员函数。
:return: Canny 算法的结果
'''
self.img = cv2.GaussianBlur(self.img, (self.Guassian_kernal_size, self.Guassian_kernal_size), 0)
self.Get_gradient_img()
self.img_origin = self.img.copy()
self.Non_maximum_suppression()
self.Hysteresis_thresholding()
return self.img
# Author: Ji Qiu (BUPT)
# filename: my_hough.py
import numpy as np
import math
class Hough_transform:
def __init__(self, img, angle, step=5, threshold=135):
'''
:param img: 输入的图像
:param angle: 输入的梯度方向矩阵
:param step: Hough 变换步长大小
:param threshold: 筛选单元的阈值
'''
self.img = img
self.angle = angle
self.y, self.x = img.shape[0:2]
self.radius = math.ceil(math.sqrt(self.y**2 + self.x**2))
self.step = step
self.vote_matrix = np.zeros([math.ceil(self.y / self.step), math.ceil(self.x / self.step), math.ceil(self.radius / self.step)])
self.threshold = threshold
self.circles = []
def Hough_transform_algorithm(self):
'''
按照 x,y,radius 建立三维空间,根据图片中边上的点沿梯度方向对空间中的所有单
元进行投票。每个点投出来结果为一折线。
:return: 投票矩阵
'''
print ('Hough_transform_algorithm')
for i in range(1, self.y - 1):
for j in range(1, self.x - 1):
if self.img[i][j] > 0:
y = i
x = j
r = 0
while y < self.y and x < self.x and y >= 0 and x >= 0:
self.vote_matrix[math.floor(y / self.step)][math.floor(x / self.step)][math.floor(r / self.step)] += 1
y = y + self.step * self.angle[i][j]
x = x + self.step
r = r + math.sqrt((self.step * self.angle[i][j])**2 + self.step**2)
y = i - self.step * self.angle[i][j]
x = j - self.step
r = math.sqrt((self.step * self.angle[i][j])**2 + self.step**2)
while y < self.y and x < self.x and y >= 0 and x >= 0:
self.vote_matrix[math.floor(y / self.step)][math.floor(x / self.step)][math.floor(r / self.step)] += 1
y = y - self.step * self.angle[i][j]
x = x - self.step
r = r + math.sqrt((self.step * self.angle[i][j])**2 + self.step**2)
return self.vote_matrix
def Select_Circle(self):
'''
按照阈值从投票矩阵中筛选出合适的圆,并作极大化抑制,这里的非极大化抑制我采
用的是邻近点结果取平均值的方法,而非单纯的取极大值。
:return: None
'''
print ('Select_Circle')
houxuanyuan = []
for i in range(0, math.ceil(self.y / self.step)):
for j in range(0, math.ceil(self.x / self.step)):
for r in range(0, math.ceil(self.radius / self.step)):
if self.vote_matrix[i][j][r] >= self.threshold:
y = i * self.step + self.step / 2
x = j * self.step + self.step / 2
r = r * self.step + self.step / 2
houxuanyuan.append((math.ceil(x), math.ceil(y), math.ceil(r)))
if len(houxuanyuan) == 0:
print("No Circle in this threshold.")
return
x, y, r = houxuanyuan[0]
possible = []
middle = []
for circle in houxuanyuan:
if abs(x - circle[0]) <= 20 and abs(y - circle[1]) <= 20:
possible.append([circle[0], circle[1], circle[2]])
else:
result = np.array(possible).mean(axis=0)
middle.append((result[0], result[1], result[2]))
possible.clear()
x, y, r = circle
possible.append([x, y, r])
result = np.array(possible).mean(axis=0)
middle.append((result[0], result[1], result[2]))
def takeFirst(elem):
return elem[0]
middle.sort(key=takeFirst)
x, y, r = middle[0]
possible = []
for circle in middle:
if abs(x - circle[0]) <= 20 and abs(y - circle[1]) <= 20:
possible.append([circle[0], circle[1], circle[2]])
else:
result = np.array(possible).mean(axis=0)
print("Circle core: (%f, %f) Radius: %f" % (result[0], result[1], result[2]))
self.circles.append((result[0], result[1], result[2]))
possible.clear()
x, y, r = circle
possible.append([x, y, r])
result = np.array(possible).mean(axis=0)
print("Circle core: (%f, %f) Radius: %f" % (result[0], result[1], result[2]))
self.circles.append((result[0], result[1], result[2]))
def Calculate(self):
'''
按照算法顺序调用以上成员函数
:return: 圆形拟合结果图,圆的坐标及半径集合
'''
self.Hough_transform_algorithm()
self.Select_Circle()
return self.circles
调用
# Author: Ji Qiu (BUPT)
# filename: main.py
import cv2
import math
from my_hough import Hough_transform
from my_canny import Canny
# np.set_printoptions(threshold=np.inf)
Path = "picture_source/picture.jpg"
Save_Path = "picture_result/"
Reduced_ratio = 2
Guassian_kernal_size = 3
HT_high_threshold = 25
HT_low_threshold = 6
Hough_transform_step = 6
Hough_transform_threshold = 110
if __name__ == '__main__':
img_gray = cv2.imread(Path, cv2.IMREAD_GRAYSCALE)
img_RGB = cv2.imread(Path)
y, x = img_gray.shape[0:2]
img_gray = cv2.resize(img_gray, (int(x / Reduced_ratio), int(y / Reduced_ratio)))
img_RGB = cv2.resize(img_RGB, (int(x / Reduced_ratio), int(y / Reduced_ratio)))
# canny takes about 40 seconds
print ('Canny ...')
canny = Canny(Guassian_kernal_size, img_gray, HT_high_threshold, HT_low_threshold)
canny.canny_algorithm()
cv2.imwrite(Save_Path + "canny_result.jpg", canny.img)
# hough takes about 30 seconds
print ('Hough ...')
Hough = Hough_transform(canny.img, canny.angle, Hough_transform_step, Hough_transform_threshold)
circles = Hough.Calculate()
for circle in circles:
cv2.circle(img_RGB, (math.ceil(circle[0]), math.ceil(circle[1])), math.ceil(circle[2]), (28, 36, 237), 2)
cv2.imwrite(Save_Path + "hough_result.jpg", img_RGB)
print ('Finished!')
运行效果
学习资源:北京邮电大学计算机视觉——鲁鹏